Smart and Connected Health
Millions are suffering from COVID-19, at AI Agora we work hard to help
​
Our mission is to offer an Artificial Intelligence Based Health Monitoring and Management system which enables hospitals to 1-monitor patients remotely while they are home, 2-to predict the disease progression, and to 3-predict patients’ medical conditions, determine the health risks continuously
​Our team at AI Agora is working hard on an Artificial Intelligence Based Remote Health Monitoring and Management system that monitors patients remotely while they are home. By predicting the disease progression, or patients’ medical conditions, this system enables hospitals and medical facilities to better manage and allocate their resources needed to fight
​
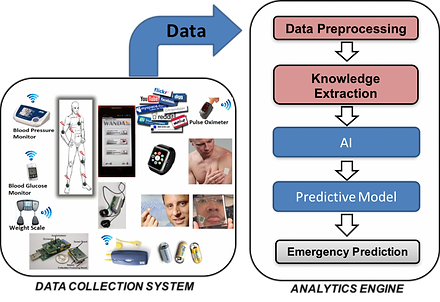

​
​
Projects
​
(1) Interactive Predictive Analytics for Enhancing Patient Adherence in Remote Health Monitoring
Although Remote Health Monitoring (RHM) systems have shown potential for improving quality of care and reducing healthcare costs, low adherence of human subjects can dramatically degrade the system efficacy. The purpose of this project is to design and
develop an interactive and human-centered framework with new data-driven techniques
and predictive analytics algorithms to enhance patients’ engagement and compliance with
RHM systems. In this project, we propose a novel interactive data-driven system for on demand
data acquisition to enhance human subjects’ adherence in a RHM system. In this
approach, we develop a predictive analytics model that attempts to predict medical
conditions with the least amount of collected data in order to reduce patients’ burden and
improve the adherence. The proposed framework includes a data-driven unit named
Interactive Learning for Data Acquisition (ILDA) for on-demand data collection in order to
enhance the prediction confidence and accuracy as needed. The ILDA automatically
decides whether it still needs to acquire additional information from some specific subjects
or not. It is responsible for evaluating the confidence of the predictions, detecting the need
to interact with specific subjects to collect new data, and identifying what additional
information should be requested from which subjects. The proposed method has been
tested and validated on two different datasets from diabetic and heart disease patients.
​
(2) Remote Health Monitoring Outcome Success Prediction Using Baseline and First Month
Intervention Data
Remote health monitoring (RHM) systems are becoming more widely adopted by clinicians
and hospitals to remotely monitor and communicate with patients while optimizing
clinician time, decreasing hospital costs, and improving quality of care. In the Women’s
heart health study (WHHS), we developed Wanda-cardiovascular disease (CVD), where
participants received healthy lifestyle education followed by six months of technology
support and reinforcement. Wanda-CVD is a smartphone-based RHM system designed to
assist participants in reducing identified CVD risk factors through wireless coaching using
feedback and prompts as social support. Many participants benefitted from this RHM
system. In response to the variance in participants’ success, we developed a framework to
identify classification schemes that predicted successful and unsuccessful participants. We
analyzed both contextual baseline features and data from the first month of intervention
such as activity, blood pressure, and questionnaire responses transmitted through the
smartphone. A prediction tool can aid clinicians and scientists in identifying participants
who may optimally benefit from the RHM system. Targeting therapies could potentially
save healthcare costs, clinician, and participant time and resources. Our classification
scheme yields RHM outcome success predictions with an F-measure of 91.9%, and
identifies behaviors during the first month of intervention that help determine outcome
success. We also show an improvement in prediction by using intervention-based
smartphone data. Results from the WHHS study demonstrates that factors such as the
variation in first month intervention response to the consumption of nuts, beans, and
seeds in the diet help predict patient RHM protocol outcome success in a group of young
Black women ages 25-45.
​
(3) A Robust Remote Health Monitoring and Data Processing System for Rural Area with Limited
Internet Access
​
Emerging technologies such as Body Sensor Networks (BSN) and Remote Health
Monitoring Systems (RHMS) allow for collecting continuous data from patients, providing
clinical interventions to improve patients’ physical and mental health, and to prevent
medically adverse events. Although RHMS have shown promises in reducing healthcare
costs and improving quality of care, designing a robust, flexible and portable RHMS that
can adequately handle technological and environmental restrictions such as data
communication disruptions caused by low-quality infrastructure is by large an open
problem.
In this study we present an end-to-end robust RHMS including body area networks for
monitoring and management of patients with HIV/AIDS. The proposed system includes an
Android application to be installed on mobile devices to collect and preprocess data from
patients, a wireless body area network to handle data communication and transmission,
and a backend cloud-based server coupled with a NoSQL database and web-portal for data
visualization and analysis.
The proposed system addresses a number of serious challenges in RHMS design including
data transmission and synchronization in a body area network with poor network
connection (e.g. in a rural area with poor or unavailable internet connection). The
proposed system is fully implemented and deployed in a large research study involving
600 women living with HIV/AIDS in rural parts of India. The results demonstrate the
effectiveness, reliability, robustness, and accuracy of the proposed RHMS.
(4) Multiple model analytics for adverse event
prediction in remote health monitoring systems
Remote health monitoring systems (RHMS) are gaining an important role in healthcare by
collecting and transmitting patient vital information and providing data analysis and
medical adverse event prediction (e.g. hospital readmission prediction). Reduction in the
readmission rate is typically achieved by early prediction of the readmission based on the
data collected from RHMS, and then applying early intervention to prevent the
readmission. Given the diversity of patient populations and the continuous nature of
patient monitoring, a single static predictive model is insu􀃞cient for accurately predicting
adverse events. To address this issue, we propose a multiple prediction modeling
technique that includes a set of accurate prediction models rather than one single
universal predictor. In this study, we propose a novel analytics framework based on the
physiological data collected from RHMS, advanced clustering algorithms and multiplemodel-
classification. We tested our proposed method on a subset of data collected
through a remote health monitoring system from 600 Heart Failure patients. Our proposed
method provides significant improvements in prediction accuracy and performance over
single predictive models.
​
(5) Context-Aware Data Analytics for Activity Recognition
Remote Health Monitoring Systems (RHMS) are gaining an important role in healthcare by
collecting and transmitting patient information and providing data analytics techniques to
analyze the collected data and extract knowledge. Physical activity recognition and indoor
localization are two of the most important concepts in assistive healthcare, where tracking
the positions, motions and reactions of a patient or elderly is required for medical
observation or accident prevention. In this study, we propose a novel context-aware data
analytics framework to classify and recognize the physical activity based on the signals
received from a worn Smart Watch, the location information of the human subject, and
advanced machine learning algorithms. In this approach, we consider the physical location
of the human subject as contextual information to improve the accuracy of the activity
classification. The hypothesis is that the location information can get involved in classifier
decision making as a prior probability distribution to help improve the accuracy of activity
recognition. The results demonstrate improvements in accuracy and performance of the
activity classification when applying the proposed method compared to conventional
classifications.
​
(6) Remote health monitoring: Predicting outcome success based on contextual features
for cardiovascular disease
Current studies have produced a plethora of remote health monitoring (RHM) systems
designed to enhance the care of patients with chronic diseases. Many RHM systems are
designed to improve patient risk factors for cardiovascular disease, including physiological
parameters such as body mass index (BMI) and waist circumference, and lipid pro_les such
as low-density lipoprotein (LDL) and high-density lipoprotein (HDL). There are several
patient characteristics that could be determining factors for a patient’s RHM outcome
success, but these characteristics have been largely unidenti_ed. In this study, we analyze
results from an RHM system deployed in a six-month Women’s Heart Health study of 90
patients and apply advanced feature selection and machine learning algorithms to identify
patients’ key baseline contextual features and build eô€ƒ ective prediction models that help
determine RHM outcome success. We introduce Wanda-CVD, a smartphone-based RHM
system designed to help participants with cardiovascular disease risk factors by motivating
participants through wireless coaching using feedback and prompts as social support. We
analyze key contextual features that secure positive patient outcomes in both physiological
parameters and lipid pro_les. Results from the Women’s Heart Health study show that
health threat of heart disease, quality of life, family history, stress factors, social support,
and anxiety at baseline all help predict patient RHM outcome success.
(7) A Framework for Predicting Adherence in
Remote Health Monitoring Systems
Remote health monitoring (RHM) systems have shown potential eô€ƒ ectiveness in disease
management and prevention. In several studies RHM systems have been shown to reduce
risk factors for cardiovascular disease (CVD) for a subset of the study participants.
However, many RHM study participants fail to adhere to the prescribed study protocol or
end up dropping from the study prior to its completion. In a recent Women’s Heart Health
study of 90 individuals in the community, we developed Wanda-CVD, an enhancement to
our previous RHM system. Wanda-CVD is a smartphone-based RHM system designed to
assist participants to reduce identi_ed CVD risk factors by motivating participants through
wireless coaching using feedback and prompts as social support. Many participants
adhered to the study protocol, however, many did not completely adhere, and some even
dropped prior to study completion. In this study, we present a framework for analyzing
baseline features to predict adherence to prescribed medical protocols that can be applied
to other RHM systems. Such a prediction tool can aid study coordinators and clinicians in
identifying participants who will need further study support, leading potentially to
participants deriving maximal bene_t from the RHM system, potentially saving healthcare
costs, clinician and participant time and resources. We analyze key contextual features that
predict with an accuracy of 85.2% which participants are more likely to adhere to the study
protocol. Results from the Women’s Heart Health study demonstrate that factors such as
perceived health threat of heart disease, and perceived social support are among the
factors that aid in predicting patient RHM protocol adherence in a group of African
American women ages 25-45.
(8) Probabilistic segmentation of time-series
audio signals using Support Vector Machines
To allow health tracking, patient monitoring, and provide timely user interventions, sensor
signals from body sensor networks need to be processed in real-time. Time subdivisions of
the sensor signals are extracted and fed into a supervised learning algorithm, such as
Support Vector Machines (SVM), to learn a model capable of distinguishing diô€ƒ erent class
labels. However, selecting a short-duration window from the continuous data stream is a
signi_cant challenge, and the window may not be properly centered around the activity of
interest. In this work, we address the issue of window selection from a continuous data
stream, using an optimized SVM-based probability model. To evaluate the eô€ƒ ectiveness of
our approach, we apply our algorithm to audio signals acquired from a wearable nutritionmonitoring
necklace. Our optimized algorithm is capable of correctly classifying 86.1% of
instances, compared to a baseline of 73% which segments the time-series data with _xedsize
non-overlapping windows, and an exhaustive-search approach with an accuracy of
92.6%.
(9) Improving compliance in remote healthcare
systems through smartphone battery
optimization
Remote health monitoring (RHM) has emerged as a solution to help reduce the cost
burden of unhealthy lifestyles and aging populations. Enhancing compliance to prescribed
medical regimens is an essential challenge to many systems, even those using smartphone
technology. In this study, we provide a technique to improve smartphone battery
consumption and examine the eô€ƒ ects of smartphone battery lifetime on compliance, in an
attempt to enhance users’ adherence to remote monitoring systems. We deploy WANDACVD,
an RHM system for patients at risk of cardiovascular disease (CVD), using a wearable
smartphone for detection of physical activity. We tested the battery optimization technique
in an in-lab pilot study and validated its eô€ƒ ects on compliance in the Women’s Heart Health
Study. The battery optimization technique enhanced the battery lifetime by 192% on
average, resulting in a 53% increase in compliance in the study. A system like WANDA-CVD
can help increase smartphone battery lifetime for RHM systems monitoring physical
activity.
(10) Anti-Cheating: Detecting Self-In􀃖icted and
Impersonator Cheaters for Remote Health
Monitoring Systems with Wearable Sensors
In remote health monitoring of patient’s physical activity, ensuring correctness and
authenticity of the received data is essential. Although many activity monitoring systems,
devices and techniques have been developed, preventing patient cheating of an activity
monitor has been a primarily unaddressed challenge across the board. Patients can
manually shake an activity monitor device (sensor) with their hand and watch their physical
activity points or rewards increase, we deô€ƒ•ne this as “self-inô€ƒ–icted” cheating. A second type
of cheating, “impersonator” cheating, is when subjects hand the activity sensor over to a
friend or second party to wear and perform physical activity on their behalf. In this study,
we propose two novel methods based on classi􀃕cation algorithms to address the cheating
problems. The 􀃕rst classi􀃕cation framework improves the correctness of our data by
detecting self-in􀃖icted cheatings. The second technique is an advanced classi􀃕cation
scheme that extracts and learns unique patient-speci􀃕c activity patterns from prior data
collected on a patient to distinguish the true subject from an impersonator. We tested our
proposed techniques on Wanda, a remote health monitoring system used in our Women’s
Heart Health study of 90 African American women at risk of cardiovascular disease. We
were able to distinguish cheating from other physical activities such as walking and
running, as well as other common activities of daily living such as driving and playing video
games. The self-in􀃖icted cheating classi􀃕er achieved an accuracy of above 90% and an AUC
of 99%. The impersonator cheater framework results in an average accuracy of above 90%
and an average AUC of 94%. Our results provide insight into the randomness of cheating
activities, successfully detects cheaters, and attempts to build more context-aware remote
activity monitors that more accurately capture patient activity.
(11) Classi􀃕cation for Big Dataset of Bioacoustic
Signals Based on Human Scoring System and
Arti􀃕cial Neural Network
In this project, we propose a method to improve sound classi􀃕cation performance by
combining signal features, derived from the time-frequency spectrogram, with human
perception. The method presented herein exploits an arti􀃕cial neural network (ANN) and
learns the signal features based on the human perception knowledge. The proposed
method is applied to a large acoustic dataset containing 24 months of nearly continuous
recordings. The results show a signi􀃕cant improvement in performance of the detectionclassi
􀃕cation system; yielding as much as 20% improvement in true positive rate for a
given false positive rate.
(12) Applying machine learning techniques to
recognize arc in vehicle 48 electrical systems
Implementing higher voltages in vehicles like 48V mild hybrid or full-hybrid enables CO2
reduction and weight savings. However, the increase in the voltage demands an accurate
and robust protection system again potential fault conditions. Series arc is one of the fault
conditions which needs to be detected and addressed before the bene_ts of using higher
voltages in vehicle can be fully realized. In this project, an e􀃞cient arc detection algorithm
is presented based on advanced machine learning algorithms to recognize series arcs in
the electrical systems of 48V vehicles. The results demonstrate that the proposed
algorithm recognizes the arcs in the signal with accuracy of more than 99% detection. The
algorithm only uses measured current and therefore, no additional wiring will be added to
the system.
(13) Framework for Domain-Agnostic Gait Cycle
Detection
The utility of wearable sensors for continuous gait monitoring has grown substantially,
enabling novel applications on mobility assessment in healthcare. Existing approaches for
gait cycle detection rely on prede_ned or experimentally tuned platform parameters and
are often platform-speci_c, parameter sensitive, and unreliable in noisy environments with
constrained generalizability. To address these challenges, we introduce Framework1, a
novel framework for reliable and platform-independent gait cycle detection. Framework
oô€ƒ ers unique features: (1) It leverages physical properties of human gait to learn model
parameters; (2) captured signals are transformed into signal magnitude and processed
through a normalized cross-correlation module to compensate for noise and search for
repetitive patterns without prede_ned parameters; (3) an optimal peak detection algorithm
is developed to accurately _nd strides within the motion sensor data. To demonstrate the
e􀃞ciency of Framework, three experiments are conducted: a clinical study including a
visually impaired group of patients with glaucoma and a control group of healthy
participants; a clinical study involving children with Rett syndrome; and an experiment
involving healthy participants. The performance of Framework is assessed under varying
platform settings and demonstrates to maintain over 93% accuracy under noisy signal,
varying bit resolutions, and changes in sampling frequency. This translates into a recall of
95.3% and a precision of 93.4%, on average. Moreover, Framework can detect strides and
estimate cadence using data from diô€ƒ erent sensors, with accuracy higher than 95% and it
is robust to random sensor orientations with a recall of 91.5% and a precision of 99.2%, on
average.
(14) Predictive Analytics to Determine the
Potential Occurrence of Genetic Disease and
their Correlation: Osteoporosis and
Cardiovascular Disease
In this project, a Predictive Analytics Model is designed, developed, and validated to
determine the risk of manifesting osteoporosis in later life using big data processing. The
proposed model leverages the novel genetic pleiotropic information in the 1,000 Genome
Project of over 2,500 individuals world-wide. Also, the mutations associated with
osteoporosis and cardiovascular disease are speci_cally analyzed. The study proposes the
automatic histogram clustering as an eô€ƒ ective and intuitive visualization method for high
dimensional dataset. The results demonstrate a signi_cant correlation between a person’s
regional background and the frequency of occurrence of the 35 Single Nucleotide
Polymorphisms (SNPs) associated with osteoporosis and/or cardiovascular disease (CVD).
Machine learning algorithms, such as Logistic Regression, Adaboost, and KNN are then
applied to predict the occurrence of 7 osteoporosis-related SNPs based on the existing
CVD-related-SNPs input. Finally, the developed model is evaluated using a separate dataset
obtained through Aô€ƒ ymetrix microarray mRNA expression signal values for the speci_c
SNP(s) in individuals with and without osteoporosis.
(15) Multi-label Classi_cation of Single and
Clustered Cervical Cells Using Deep
Convolutional Networks
Cytology-based screening through the Papanicolaou test has dramatically decreased the
incidence of cervical cancer. Convolutional neural networks (CNN) have been utilized to
classify cancerous cervical cytology cells but primarily focused on pre-processed nuclear
details for a single cell and binary classi_cation of normal versus abnormal cells. In this
study, we developed a novel system for multiple label classi_cation with a focus on both
nucleus and cytoplasm of single cells and cell clusters. In this retrospective study, we
digitalized cervical cytology slides from 104 patients. Based upon the Bethesda system, the
established criteria for diagnosing cervical cytology, cells of interest were categorized. With
10-fold cross validation, our CNN algorithm demonstrated 84.5% overall accuracy, 79.1%
sensitivity, 89.5% speci_city for normal versus abnormal. For 3 level classi_cation of
normal, low-grade, and high-grade, CNN demonstrated 76.1% overall accuracy. Results
show promise on the utility of CNNs to learn cervical cytology.
(16) Predicting Glucose Levels in Patients with
Type1 Diabetes Based on Physiological and
Activity Data
Managing blood glucose levels for type 1 diabetes patients is an absolute necessity to
better glycemic control. In this study, we present a predictive model that uses physiological
measurements and physical activity to predict continuous glucose levels and help patients
reduce and prevent hyperglycemia and hypoglycemia exposure, conditions that are
harmful to patient health.
The data of this study includes 4 months of physiological measurements, physical activity,
and nutrition information collected from 93 patients with diabetes using the Medtronic
MiniMed™ 530G insulin delivery system with Enlite™ sensor. After data preprocessing,
missing value imputation, feature extraction, and feature selection, a set of 180 features
were derived to represent the raw data. Then, an appropriate predictive model was
developed based on machine-learning algorithms to predict continuous glucose levels. The
prediction accuracy and error have been calculated to evaluate the performance of the
system. The results demonstrated that the predicted glucose levels closely followed the
actual sensor glucose (SG) values measured by subcutaneous glucose sensor.
(17) A novel fast solving method for targeted
drug-delivery capsules in the gastrointestinal
tract
As an innovative technique without cable connection, targeted drug-delivery capsules
improve diagnostic and therapeutic capabilities in the gastrointestinal (GI) tract. To fast
track targeted drug-delivery capsules in the GI tract, a tracking method based on the
multiple alternating magnetic sources with adaptive adjustment of the excitation intensity
has been investigated. The functional prototype of the tracking system has been
developed. The tracking model between the magnetic ô€ƒ•eld strength and the capsule’s
location has been established, which shows a nonlinear equation group with multiple local
extremum. Particularly, an improved back-propagation (BP) neural network by particle
swarm optimization (PSO) is investigated to solve the tracking problem in real time. The
PSO is introduced at an early stage to optimize the weights and thresholds of the BP neural
network to improve the generalizability and global search ability. Consequently, the
Levenberg-Marquardt (LM) algorithm is used as the learning rule to obtain a higher
accuracy and convergence rate. The performance on the PSO-BP neural network is
experimentally analyzed by comparing it with the standard BP network and the LM-BP
network. The tracking experiments show that the PSO-BP neural network can solve the
tracking problem successfully. The PSO-BP network can get the solution faster than
iterative search algorithms.
(18) E􀃞ciency Optimization of Deep Workout
Recognition with Accelerometer Sensor for a
Mobile Environment
Recent advancements in deep learning have created numerous possibilities for real-world
application, among which the recognition of human motions with sensors. We employed a
convolutional neural network (CNN) to process workout motion data to solve the
segmentation and recognition problem. We focused on deploying the network architecture
in a mobile environment characterized by limited resources. Our experimental results
were promising in terms of both segmentation and recognition. Furthermore, we analyzed
the performance correlation between sampling the rate and recognition rate. This result
indicated 55Hz to provide an appropriate amount of information for workout motion. Then
we investigated the computational cost and memory space usage of the hyper-parameter
selection. The experimental results implied that appropriate hyper-parameter selection
could reduce the computational burden on the mobile environment. Subsequently, we
suggest an e􀃞ciency score regarding the computational cost, memory usage, and the
recognition rate. Our suggested e􀃞ciency score shows that our method could 􀃕nd hyperparameters
that oô€ƒ er minimum loss of accuracy and are computationally inexpensive with
optimal utilization of memory space.
(19) Analyzing the Mutation Frequencies and
Correlation of Genetic Diseases in Worldwide
Populations Using Big Data Processing,
Clustering, and Predictive Analytics
In this study, we utilize Big Data Processing and develop Predictive Analytics Models to
examine and analyze mutations associated with osteoporosis and cardiovascular disease.
The dataset consists of the genomic information of over 2,500 individuals. The genomic
data was collected from all around the world. The data visualization allowed us to see
geographical/regional clustering patterns in the above-mentioned speci􀃕c mutations. The
visualized data clearly shows a high correlation between a person’s regional background
and the occurrence of the 35 single nucleotide polymorphisms (SNPs). The 35 SNPs are
speci􀃕cally associated with osteoporosis and/or cardiovascular disease (CVD). A predictive
analytics model was developed based on machine learning algorithms to predict the risk of
an individual manifesting osteoporosis in later life. The results of this predictive model
con􀃕rmed the links between osteoporosis and Cardiovascular related parameters such as
High-Density Lipoprotein (HDL) and Systolic Blood Pressure (SBP), as determined by the
preceding studies.
(20) A 􀃖exible data-driven comorbidity feature
extraction framework
Disease and symptom diagnostic codes are a valuable resource for classifying and
predicting patient outcomes. In this study, we propose a novel methodology for utilizing
disease diagnostic information in a predictive machine learning framework. Our
methodology relies on a novel, clustering-based feature extraction framework using
disease diagnostic information. To reduce the data dimensionality, we identify disease
clusters using co-occurrence statistics. We optimize the number of generated clusters in
the training set and then utilize these clusters as features to predict patient severity of
condition and patient readmission risk. We build our clustering and feature extraction
algorithm using the 2012 National Inpatient Sample (NIS), Healthcare Cost and Utilization
Project (HCUP) which contains 7 million hospital discharge records and ICD-9-CM codes.
The proposed framework is tested on Ronald Reagan UCLA Medical Center Electronic
Health Records (EHR) from 3041 Congestive Heart Failure (CHF) patients and the UCI 130-
US diabetes dataset that includes admissions from 69,980 diabetic patients. We compare
our cluster-based feature set with the commonly used comorbidity frameworks including
Charlson’s index, Elixhauser’s comorbidities and their variations. The proposed approach
was shown to have signi_cant gains between 10.7-22.1% in predictive accuracy for CHF
severity of condition prediction and 4.65-5.75% in diabetes readmission prediction.
(21) A data-driven feature extraction framework
for predicting the severity of condition of
congestive heart failure patients
In this study, we propose a novel methodology for utilizing disease diagnostic information
to predict severity of condition for Congestive Heart Failure (CHF) patients. Our
methodology relies on a novel, clustering-based, feature extraction framework using
disease diagnostic information. To reduce the dimensionality, we identify disease clusters
using cooccurrence frequencies. We then utilize these clusters as features to predict
patient severity of condition. We build our clustering and feature extraction algorithm
using the 2012 National Inpatient Sample (NIS), Healthcare Cost and Utilization Project
(HCUP) which contains 7 million discharge records and ICD-9-CM codes. The proposed
framework is tested on Ronald Reagan UCLA Medical Center Electronic Health Records
(EHR) from 3041 patients. We compare our cluster-based feature set with another that
incorporates the Charlson comorbidity score as a feature and demonstrate an accuracy
improvement of up to 14% in the predictability of the severity of condition.
(22) Accurate tumor localization and tracking in
radiation therapy using wireless body sensor
networks
Radiation therapy is an eô€ƒ ective method to combat cancerous tumors by killing the
malignant cells or controlling their growth. Knowing the exact position of the tumor is a
very critical prerequisite in radiation therapy. Since the position of the tumor changes
during the process of radiation therapy due to the patients’ movements and respiration, a
real-time tumor tracking method is highly desirable in order to deliver a su􀃞cient dose of
radiation to the tumor region without damaging the surrounding healthy tissues. In this
study, we develop a novel tumor positioning method based on spatial sparsity. We
estimate the position by processing the received signals from only one implantable RF
transmitter. The proposed method uses a smaller number of sensors compared to
common magnetic transponder-based approaches. The performance of the proposed
method is evaluated in two diô€ƒ erent cases: (1) when the tissue con_guration is perfectly
determined (acquired beforehand by MRI or CT) and (2) when there are some uncertainties
about the tissue boundaries. The results demonstrate the high accuracy and performance
of the proposed method, even when the tissue boundaries are imperfectly known.
(23) Accurate Localization of In-Body Medical
Implants Based on Spatial Sparsity
Wearable and implantable wireless communication devices have in recent years gained
increasing attention for medical diagnostics and therapeutics. In particular, wireless
capsule endoscopy has become a popular method to visualize and diagnose the human
gastrointestinal tract. Estimating the exact position of the capsule when each image is
taken is a very critical issue in capsule endoscopy. Several approaches have been
developed by researchers to estimate the capsule location. However, some unique
challenges exist for in-body localization, such as the severe multipath issue caused by the
boundaries of diô€ƒ erent organs, inconsistency of signal propagation velocity and path loss
parameters inside the human body, and the regulatory restrictions on using highbandwidth
or high-power signals. In this study, we propose a novel localization method
based on spatial sparsity. We directly estimate the location of the capsule without going
through the usual intermediate stage of _rst estimating time-of-arrival or received-signal
strength, and then a second stage of estimating the location. We demonstrate the accuracy
of the proposed method through extensive Monte Carlo simulations for radio frequency
emission signals within the required power and bandwidth range. The results show that
the proposed method is eô€ƒ ective and accurate, even in massive multipath conditions.
(24) Indoor Localization, Tracking and Fall
Detection for Assistive Healthcare Based on
Spatial Sparsity and Wireless Sensor Network
Indoor localization and fall detection are two of the most paramount topics in assistive
healthcare, where tracking the positions and actions of the patient or elderly is required
for medical observation or accident prevention. Most of existing indoor localization
methods are based on estimating one or more location-dependent signal parameters.
However, some challenges caused by the complex scenarios within a closed space
signi_cantly limit the applicability of those existing approaches in an indoor environment,
such as the severe multipath eô€ƒ ect. In this study, the authors propose a new one-stage,
three-dimensional localization method based on the spatial sparsity in the x-y-z space. The
proposed method is not only able to estimate and track the accurate positions of the
patient, but also capable to detect the falls of the patient. In this method, the authors
directly estimate the location of the emitter without going through the intermediate stage
of TOA or signal strength estimation. The authors evaluate the performance of the
proposed method using various Monte Carlo simulation settings. The results show that the
proposed method is a very accurate even with a small number of sensors and ii very
eô€ƒ ective in addressing the multi-path issues.
​
(25) Robustness analysis of sparsity-based tumor
localization under tissue con_guration
uncertainty
Knowing the exact position of the tumor is a very critical prerequisite in radiation therapy.
Since the position of the tumor changes because of respiration or patient movements, a
real-time tumor tracking method must be applied during the process of radiation therapy
in order to deliver a su􀃞cient dose of radiation to the tumor region without damaging the
surrounding healthy tissues. In this study, we develop a novel tumor positioning method
based on spatial sparsity and then we investigate the sensitivity of this method to the
uncertainty of tissue con_guration. The proposed method is easier to implement, noniterative,
faster and more accurate compared to common magnetic transponder-based
approaches. The performance of the proposed method is evaluated in two diô€ƒ erent cases:
(1) when the tissue con_guration is perfectly determined (acquired beforehand by MRI or
CT) and (2) when there are some uncertainties about the tissue boundaries. The results
demonstrate the satisfactory accuracy and high performance of the proposed method,
even when the tissue boundaries are imperfectly known.
​
(26) A novel method for tumor localization and
tracking in radiation therapy
Because the position of a tumor changes during radiation therapy (because of respiration
or patient movements), real-time tumor tracking is necessary during radiation therapy in
order to deliver a su􀃞cient dose of radiation to the tumor without damaging the
​
​
surrounding healthy tissues. In this study, we propose a novel tumor positioning method
based on spatial sparsity. We estimate the position by processing the received signals from
only one implantable RF transmitter. The method is easier to implement, non-iterative,
faster and more accurate compared to common magnetic transponder-based methods.
We evaluate the performance of the proposed method using Monte-Carlo simulation.
​
(27) A novel method for medical implant in-body
localization
Wireless communication medical implants are gaining an important role in healthcare
systems by controlling and transmitting the vital information of the patients. Recently,
Wireless Capsule Endoscopy (WCE) has become a popular method to visualize and
diagnose the human gastrointestinal (GI) tract. Estimating the exact location of the capsule
when each image is taken is a very critical issue in capsule endoscopy. Most of the
common capsule localization methods are based on estimating one or more locationdependent
signal parameters like TOA or RSS. However, some unique challenges exist for
in-body localization due to the complex nature within the human body. In this study, we
propose a novel one-stage localization method based on spatial sparsity in 3D space. In
this method, we directly estimate the location of the capsule (as the emitter) without going
through the intermediate stage of TOA or signal strength estimation. We evaluate the
performance of the proposed method using Monte Carlo simulation with an RF signal
following the allowable power and bandwidth ranges according to the standards. The
results show that the proposed method is very eô€ƒ ective and accurate even in massive
multipath and shadowing conditions.
​
(28) Spatial sparsity based indoor localization in
wireless sensor network for assistive healthcare
Indoor localization is one of the key topics in the area of wireless networks with increasing
applications in assistive healthcare, where tracking the position and actions of the patient
or elderly are required for medical observation or accident prevention. Most of the
common indoor localization methods are based on estimating one or more locationdependent
signal parameters like TOA, AOA or RSS. However, some di􀃞culties and
challenges caused by the complex scenarios within a closed space signi_cantly limit the
applicability of those existing approaches in an indoor assistive environment, such as the
well-known multipath eô€ƒ ect. In this study, we develop a new one-stage localization method
based on spatial sparsity of the x-y plane. In this method, we directly estimate the location
of the emitter without going through the intermediate stage of TOA or signal strength
estimation. We evaluate the performance of the proposed method using Monte Carlo
simulation. The results show that the proposed method is (i) very accurate even with a
small number of sensors and (ii) very e_ective in addressing the multi-path issues.
​